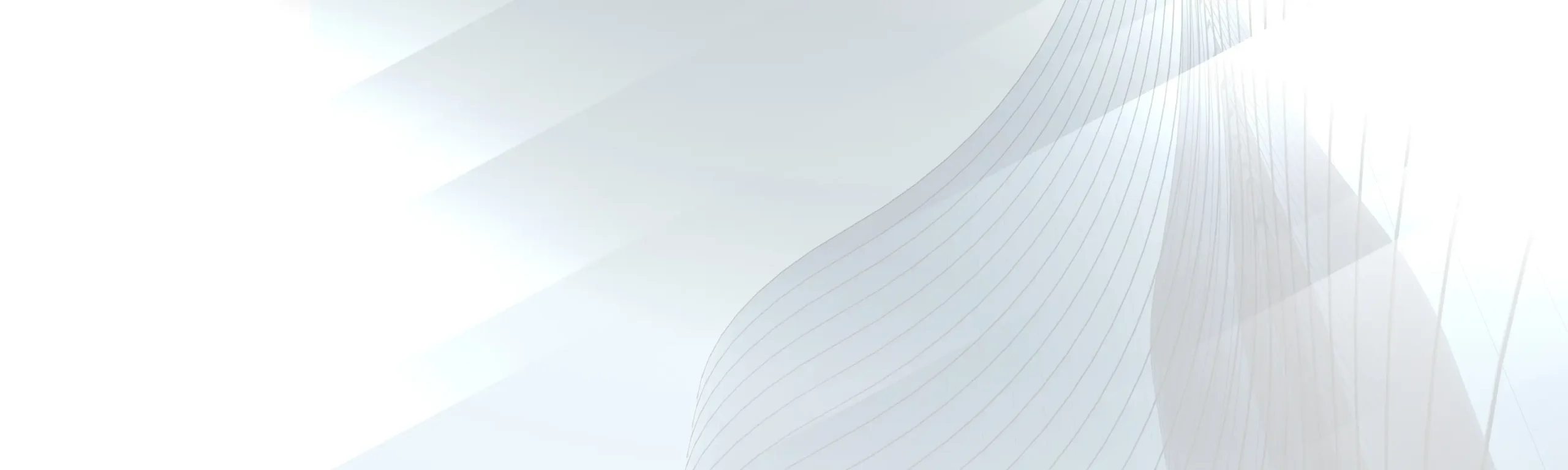
Regulating the Wicked: Resilience for AI
A tale of competing regulatory models
The explosion of generative artificial intelligence applications, like ChatGPT, Stable Diffusion, and Sora, producing expert computer code, visually stunning images, and human-like conversations, has fueled scenarios of job loss, deepfakes, intellectual property infringements, and at the extreme human extinction. At the same time, massive increases in productivity could spur company and country growth. For example, Bank of America estimates that AI will contribute $15 trillion to the global economy by 2030. Not surprisingly, regulators are busy. According to the Stanford AI Index Report, at least 123 AI-related bills were passed by 2023. But what regulation best addresses the challenge of AI?
Analysts sometimes answer this by classifying country regulatory models by the level of hard and soft regulations and whether they cover specific sectors or the entire economy (Figure 1). Of course, these models may change. For example, the USA Executive Order on AI in October 2023 is a federal government effort to provide safety and security standards and steer the USA toward harder restrictions. Analysts then weigh tradeoffs – the USA is pro-innovation, the EU is citizen-centric, and China is about state control.
Figure 1: Country Regulatory Models

AI is the most wicked of all the problems
While helpful in understanding regulatory responses, this framing does not identify what regulations best address AI’s unique characteristics. Instead, comparing AI to other wicked problems like climate change, nuclear weapons proliferation, and space debris, by degrees of consensus around the issue and ability to control it, can identify the severity of the challenge and the regulations needed. By this measure, AI is quite wicked (Figure 2).
Figure 2: Ease of regulating wicked problems, adapted from Nancy Roberts

Like other wicked problems, AI develops rapidly, has a broad impact on the economy, society, and government, requires significant expertise to truly grasp it, and has a highly uncertain risk and reward tradeoff. Unlike other problems though, there is limited consensus: many competing government and company interests hinder even defining a common problem to solve, let alone a joint solution. This is not surprising. The upside of AI is so significant that caution about downside risks has not led to any significant pauses in development. Additionally, AI changes so rapidly that regulators are hard-pressed to keep up. In 10 years, machines went from underperforming to beating humans in language and image recognition tests, according to studies reported by Max Roser in 2021.
AI offers points where regulators can intervene to control. Building cutting-edge AI models requires expertise, advanced materials like microprocessors, immense processing power, and funding that may reach above $100 million to develop high-end models, such as GPT-4. But this is changing. Costs for developing and running even the most sophisticated models are decreasing. AI models and applications are also released as open source. The most prominent may be Meta Llama 2, though others, like Google Gemma, are being launched. The noted AI model repository Hugging Face has almost 200,000 AI models for download and modification in its transformer library as of January 2024. The most popular – a speech recognition model – has over 55 million downloads.
The 3 Rs of resilience to address AI
Low consensus and control problems require resilience-focused regulations (Figure 3). Competing interests and rapid technology change make focusing on preventative and soft law regulations difficult. Pure liability-focused regulation may require too much control to set and enforce. Instead, resilience-focused regulations should be permissive, letting AI technologies advance and spread while reinforcing institutions to develop principles, standards, processes, and capabilities to head off problems before they form. These regulations are also backwards- looking, meaning they respond quickly to problems and promote recovery from any incident.
Figure 3: Regulatory responses to wicked problem domains, adapted from Marchant

Resilience regulations require capable institutions, including international organisations, national governments, companies, civil society, and people, to exhibit three features:
- Reinforce institutions to head off incidents: Integrating principles, standards, processes, and capabilities that incentivise developing, using, and testing AI systems that proactively identify and mitigate risks is the foundation to prevent AI incidents.
- Respond to risks quickly to stop incidents: Incidents like IP theft, critical infrastructure shutdowns, deepfakes influencing elections, or unforeseen ones may happen, and institutions should have the authority, processes, and capabilities to detect and stop them.
- Recover from risks by minimising damage and compensating victims. Post-incident, ensuring that victims, companies, organisations, and people are compensated will ensure trust in the use of AI and will not lead to calls for stopping the use of AI or other drastic measures.
Realising these features requires institutions to take specific regulatory actions, as per Figure 4.
Figure 4: Resilience regulatory actions across institutions

Resilience as the way forward
Already, resilience regulations are emerging. The OECD has an AI incident observatory and classifies AI systems by risk. The EU’s AI system risk categorisation, the USA’s 2023 Executive Order on AI calling for partnerships with AI companies to report red team results, and China’s algorithm registry and company security self-assessment all have resilience regulations embedded to different extents. Companies like OpenAI, Microsoft, and Google have set technical standards and even published them, such as Microsoft’s Responsible AI Standard. Three additional actions can drive resilience forward:
- First, institute binding principles and minimum technical standards for AI, which an institution similar to the IAEA can monitor.
- Second, it provides more access and resources for civil society and people to use data training sets and computing power to develop and test models.
- Third, the recovery regulations associated with resilience should be structured. This may be the most challenging because it can imply liability for incidents. However, without clear liability and compensation standards, AI companies may be reluctant to share information for fear of being later held liable.
Alternatively, regulators could increase consensus and control to move away from resilience regulations. It is a natural strategy: armies seek to change the balance of power to win in war, and companies seek to gain monopoly power. But changing these parameters is hard. Forty-four years have passed since the first mention of climate change at the UN’s First Earth Summit in 1972, when advocates and scientists helped set the binding targets of the 2016 Paris Agreement. But the world does not have that long to wait. If AI causes a banking collapse, knocks out critical infrastructure, or causes some other widespread societal harm, governments may be pressured to impose severe authoritarian regulations regardless of feasibility or lost benefits. There is no time like the present, and the upcoming AI Safety Summits in South Korea and France in 2024 will provide venues for regulators to move more explicitly toward resilience.
Author
Contact us
Subscribe to our newsletter
Subscribe now to receive our latest news